News Story
Advancing Autonomous Systems Education and Research
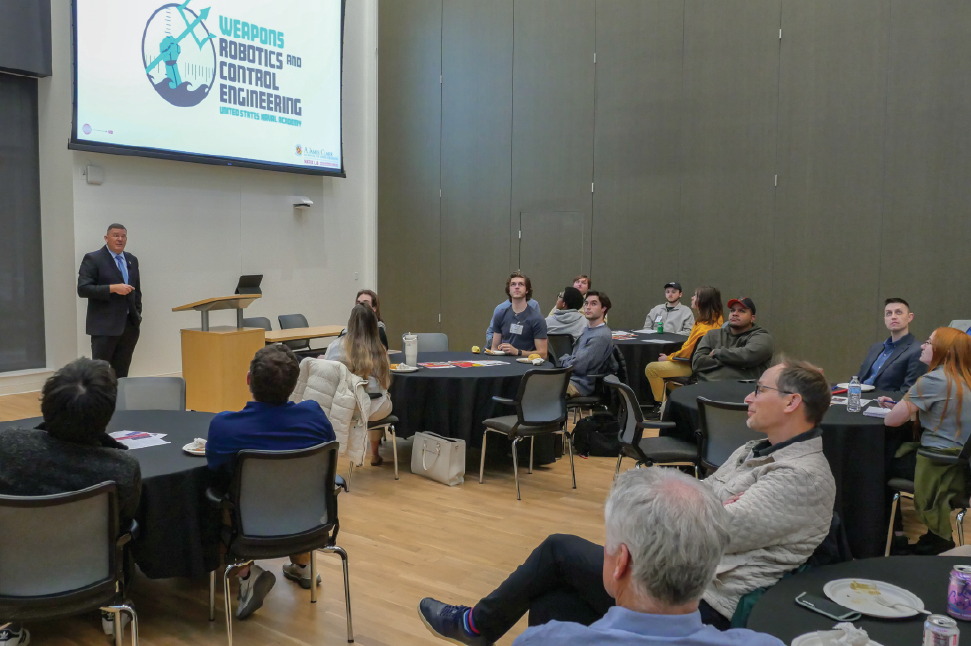
Dr. Costello presents research at March 5 Evening@SMART event
As the MATRIX Lab’s Director: Test and Evaluation of Autonomous Systems, Dr. Donald “Bucket” Costello III is spearheading both education and research initiatives.
In his March 5 Evening@SMART presentation, Dr. Costello outlined his efforts to advance the autonomy/AI field. He’s helping to develop a curriculum focused on educating students and professionals on how to ensure autonomous technologies will do what they are expected to do. He’s also working on several research projects focused on optimizing autonomous systems in order to make them as reliable as possible, which will be required for certification officials to adopt autonomous behavior.
Testing and Evaluating Autonomy
Autonomous systems, ranging from self-driving cars to robotic vacuum cleaners, are becoming more prevalent in our everyday lives. The test and evaluation (T&E) process helps ensure that these technologies are effective and safe. The data gained during this process is used by certification officials during their risk-based fielding decision.
In his research, Dr. Costello examines T&E-focused frameworks, methodologies, and approaches. He will pass on his knowledge through a T&E of autonomous systems education curriculum.
Participants will learn what to consider when planning T&E, even how to design their own tests. They’ll develop the skills they need to make good judgements and quick decisions during the T&E process.
He envisions the program, entitled Development, Test & Evaluation, Verification & Validation (DTEVV) of Autonomous Systems, will entail both a graduate certificate and a master’s degree through the University of Maryland. The program is designed for both working professionals and full-time students with the first class being offered in the Fall of 2025. This program will increase the number of DTEVV professionals, as well as increase the knowledge of the current DTEVV workforce.
Refueling Aircrafts Autonomously
One of the main thrusts of Dr. Costello’s research is to establish standards and methods of compliance for autonomous systems to complete tasks currently reserved for fully qualified humans. Over the past four years he has used the Autonomous Aerial Refueling of an Uncrewed Aerial System (UAS) as a use case to help advance this thrust.
The United States Navy (USN) intends to increase the number of uncrewed systems in its inventory, but these systems need to be able to refuel autonomously in mid-air. This task is challenging because the refueling drogue moves unpredictably in the environment.
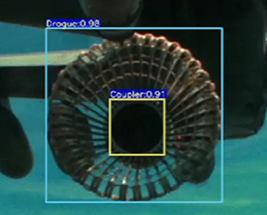
Figure 1: Image depicting the results of a DNN trained on KC-130 flight test data properly identifying the drogue and coupler behind a tanker configured F/A-18. (Mwaffo, V., Miller, D., and Costello, D., (2023). "Assessing the Predictive Performance of Two DNN Models: A Comparative Analysis to Support Reusing Training Weights for Autonomous Aerial Refueling Missions," IEEE Access, vol. 11, pp. 92070-92079, https://doi.org/10.1109/ACCESS.2023.3308822)
Machine Learning (ML) algorithms can be used to detect and track this equipment in order to determine the drogue’s exact location and pass that information to the guidance system of the UAS. This process will take place in such a way that there is no way to guarantee a human can provide oversite. This means that the UAS will have to complete the aerial refueling task autonomously (without a human in or on the loop).
Researchers, including Dr. Costello, are working on training deep neural network (DNN) models to determine standards that certification officials can use to allow the ML algorithm to make safety-critical decisions without human oversight. Figure 1 depicts a DNN trained on KC-130 imagery tracking an aerial refueling drogue behind a F/A-18.
USN UAS need to be able to distinguish between friendly vessels and enemy ships so they can safely refuel and exchange data. However, they may be operating in challenging maritime environments without a global positioning system or radio communication. Because of this, they need reliable vision to correctly identify which vessels are safe to land on. Many naval ships look very similar though, so it can be difficult for systems to tell the difference, especially in varying lighting and different environmental conditions.
Another one of Dr. Costello’s research thrusts is addressing all these concerns by equipping these systems with computer vision. Researchers train a DNN using real-world footage. A state-of-the-art object detection algorithm is used to detect and classify ships. This method makes models highlight effective at distinguishing between ship types.
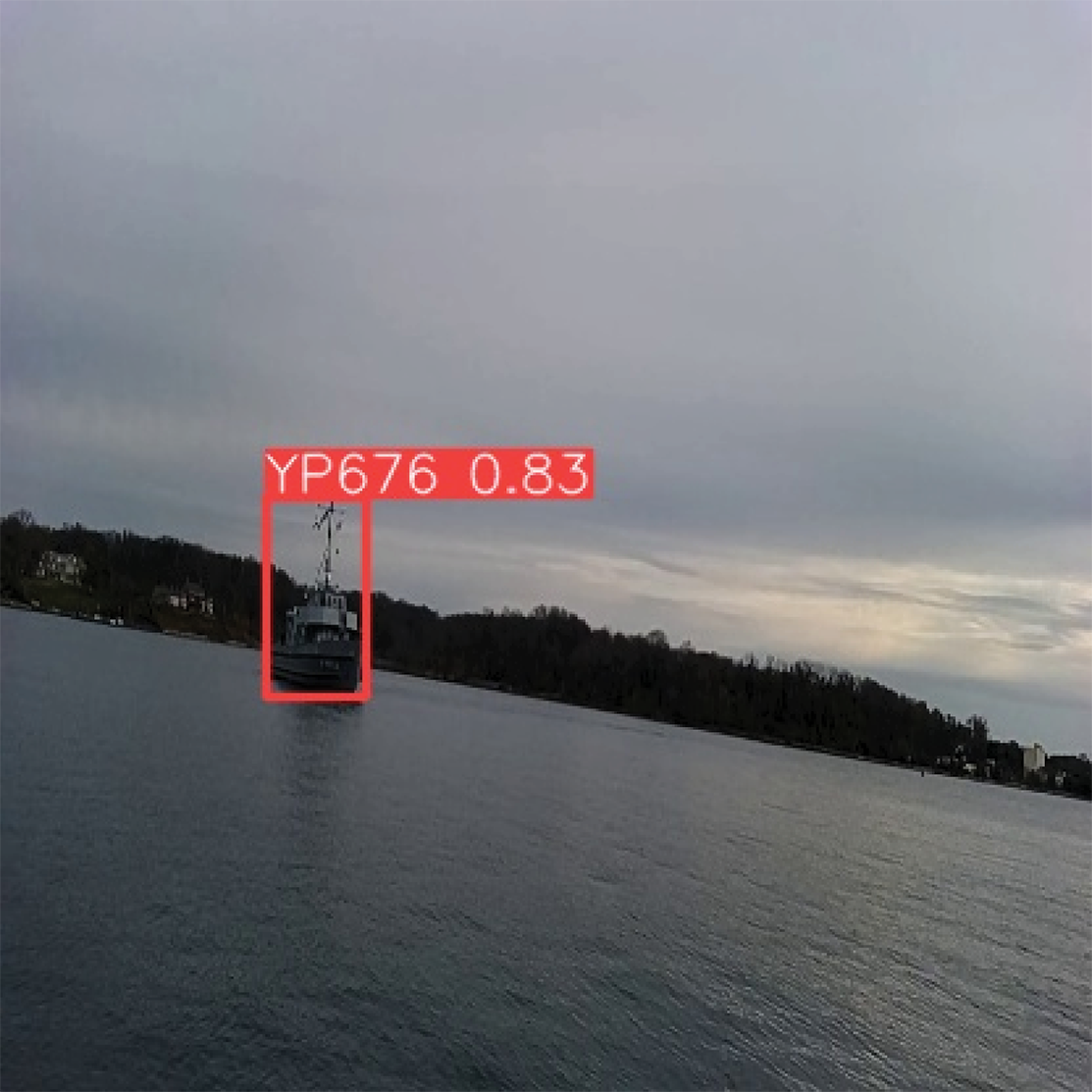
Figure 2: DNN properly identifying a naval vessel while it was underway. (McCormick, S., Richez, A., Mwaffo, V., Costello, D. (2024). “Discriminating Similar Naval Vessels Using YOLOv8 Deep Neural Network.” IEEE Access, 12, 99163-99174. 1–1. https://doi.org/10.1109/ACCESS.2024.3483014)
Enabling UAS to accurately identify ships without human input saves time, effort, and resources, efficiently advancing autonomous naval operations. Figure 2 depicts a DNN tracking a naval vessel while underway.
Since starting at the University of Maryland in 2024, Dr. Costello has published multiple peer-reviewed journal articles and conference papers, highlighting both his research thrusts and the upcoming DTEVV of Autonomous Systems. You can view those articles and papers on Dr. Costello’s faculty profile.
Next Evening@SMART
You can catch our next Evening@SMART presentation on April 2, 2025. Dr. Sanghamitra Dutta, an Assistant Professor with the Department of Electrical and Computer Engineering at the University of Maryland, will be presenting. She’ll be discussing how to ensure machine learning algorithms are explainable and trustworthy, especially in high-stakes situations. She will showcase several explanation strategies deep-rooted in mathematical foundations. Learn more and register now on the Evening@SMART web page.
Published March 24, 2025